学术报告时间:2022年11月12日(周六)09:00-11:00
学术报告地址:腾讯会议(543-612-4449)
本次人工智能学院邀请海外专家举办学术报告会议,主题为“Predicting Isoform Functions via Deep Learning and Refinement of Interaction Networks(基于深度学习及蛋白质相互作用网络的基因亚型功能预测)”。本次报告邀请美国加州大学河滨分校Tao Jiang(姜涛)教授主讲。
报告内容及摘要:Alternative splicing generates multiple isoforms from a single gene, greatly increasing the functional diversity of a genome. Although gene functions have been well studied, little is known about the specific functions of isoforms, making accurate prediction of isoform functions highly desirable. In this talk, we present two recent approaches to predict isoform functions. The first method, DIFFUSE, integrates isoform sequences and expression profiles to systematically predict isoform functions, by combining the power of deep learning and probabilistic graphical models. The second method, FINER, jointly predicts isoform functions and isoform-isoform interactions through the introduction of a unified learning objective, enabling the two tasks to benefit from each other.
Our extensive computational experiments demonstrate that these methods could effectively predict the functions of isoforms and genes with an accuracy significantly higher than the state-of-the-art methods. We further validate the prediction results by analyzing the correlation between functional similarity, sequence similarity, expression similarity, and structural similarity, as well as the consistency between the predicted functions and well-studied functional sequence features, tissue specificity and subcellular localization of isoforms in the literature.
主讲人简介:Tao Jiang received B.S. in Computer Science and Technology from the University of Science and Technology of China, Hefei, in July 1984 and Ph.D. in Computer Science from University of Minnesota in Nov. 1988. He was a faculty member at McMaster University, Hamilton, Ontario, Canada during Jan. 1989 - July 2001 and is now Distinguished Professor of Computer Science and Engineering at University of California - Riverside (UCR). He is also a member of the UCR Institute for Integrative Genome Biology, a member of the Center for Plant Cell Biology and Distinguished Visiting Professor at Tsinghua University. Tao Jiang's recent research interest includes combinatorial algorithms, computational molecular biology, bioinformatics, and computational aspects of information retrieval. He is a fellow of the Association for Computing Machinery (ACM) and of the American Association for the Advancement of Science (AAAS), and held a Presidential Chair Professor position at UCR during 2007-2010. He has published over 300 papers in computer science and bioinformatics journals and conferences, and won several best paper/poster awards. More information about his work can be found at http://www1.cs.ucr.edu/~jiang
欢迎各位老师与其他感兴趣的同学积极参与。
联系人:许翱宇
电 话:0411-84727806
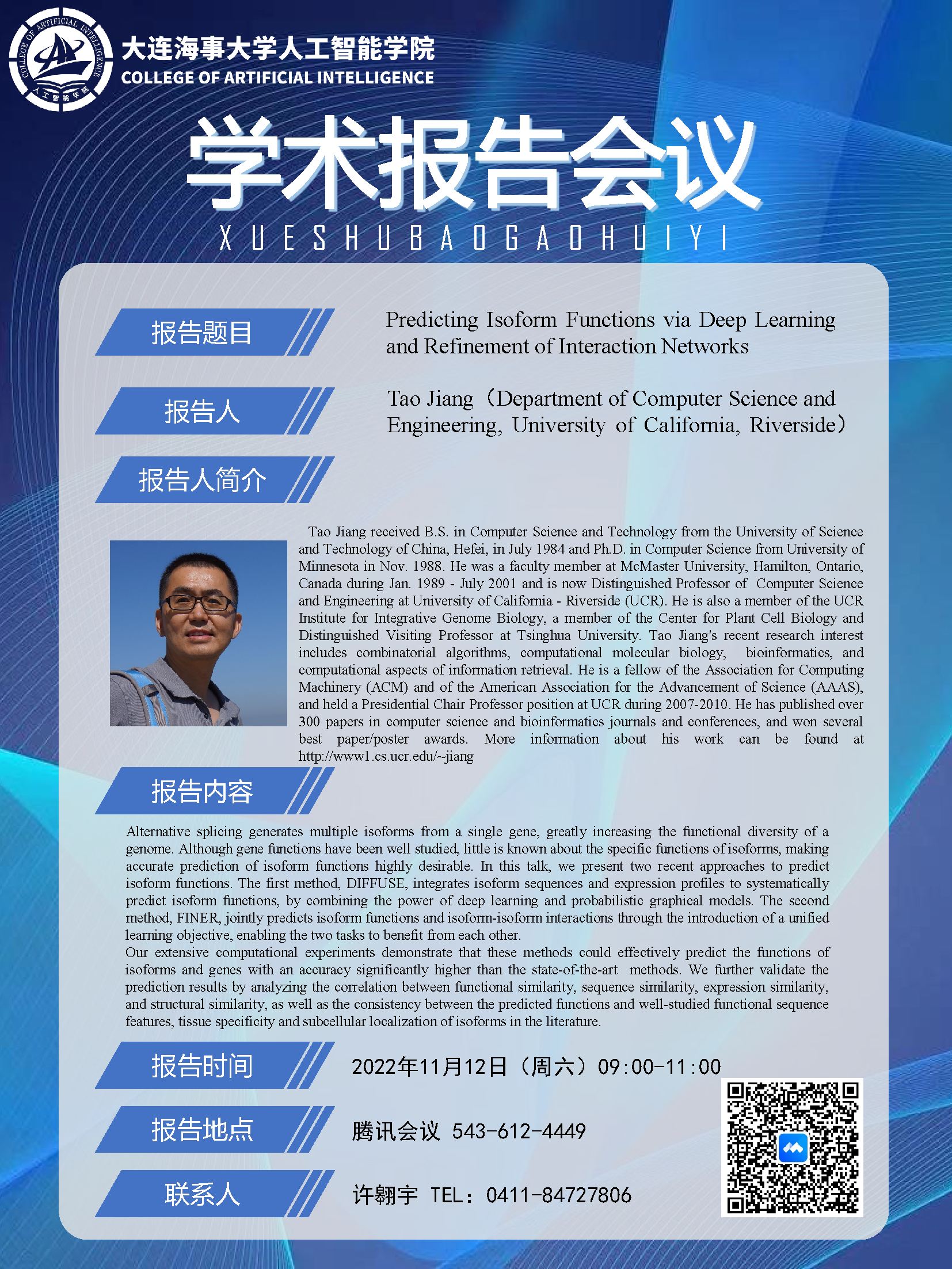
人工智能学院
2022年11月10日